Authors: Manuel Mendoza Hurtado, Domingo Ortiz Boyer
Abstract:
The identification of significant places using mobility data is an interesting field that benefits from the expansion of mobile technologies usage, which generates enormous amounts of data that have a wide variety of uses.
This paper presents a study for different multi-label classification techniques for the area identification field.
The multi-label approach is novel and more suitable than just assigning one class to each zone, since there are areas that can be both residential and working places along with other meaningful classes. Using passive mobile positioning data offers a powerful tool to study the geography and mobility of the population. We will use a mobility dataset from the city of Milan to achieve this, manually labeling a 20-by-20 subgrid of the city to make the experiments that will be evaluated in order to see which classifier is best predicting the labels, and later the results will be extrapolated to the whole grid in order to predict the classes for the city with the method that performed best.
The results have shown that this approach is valid to predict a number of meaningful labels, obtaining best results for this specific data source using Binary Relevance with a kNN classifier and Label Powerset with Random Forests, and the results can be extrapolated to predict the classes for the whole city by previously training the model with a small subset. The combination of labels obtained for each grid gives a detailed overview of the different areas of the city.
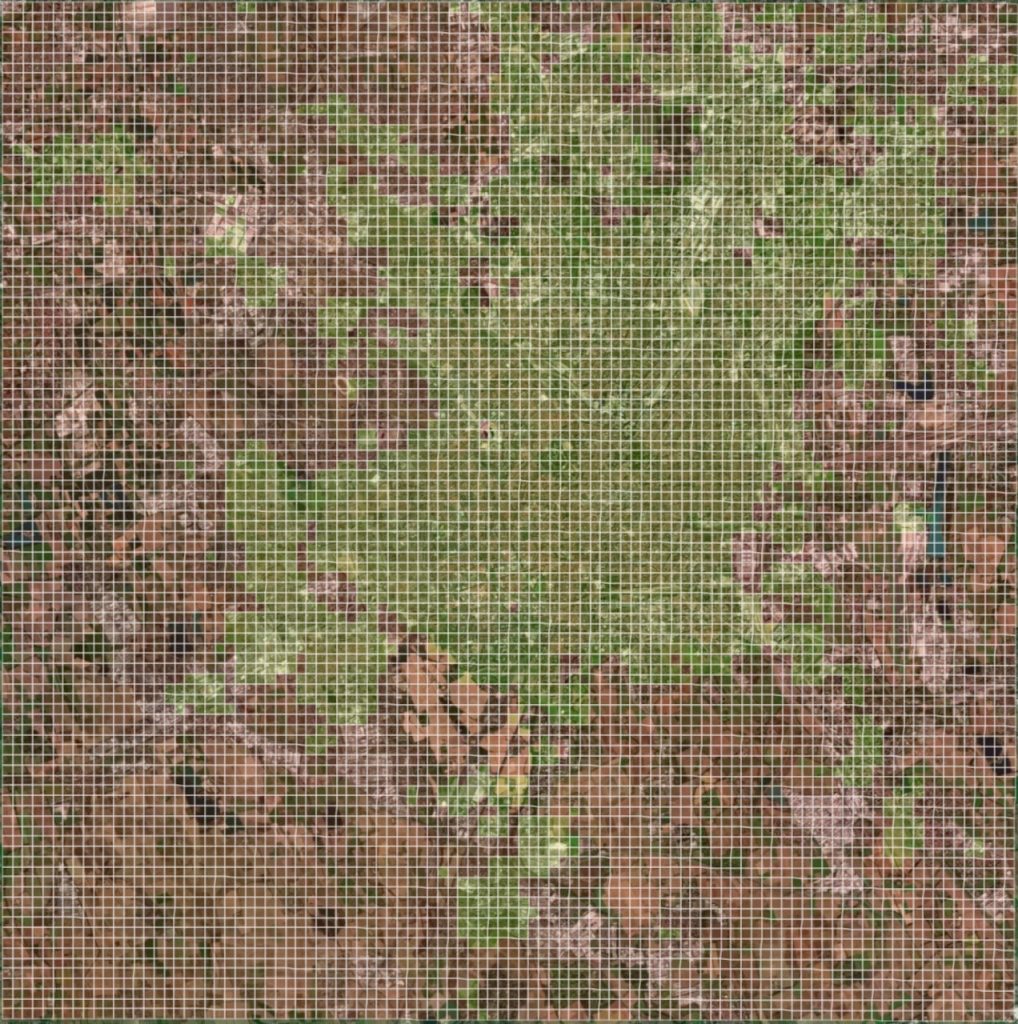
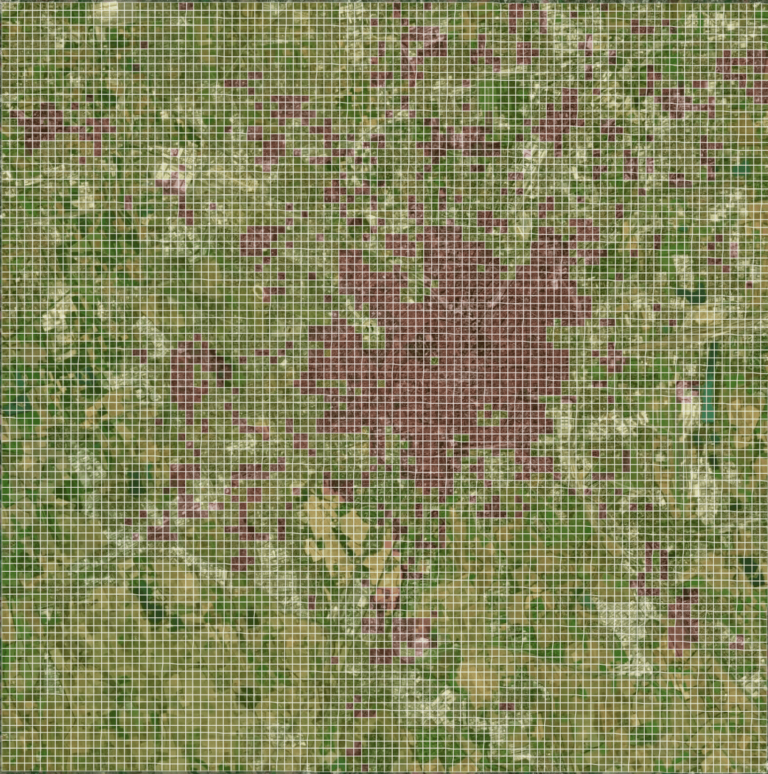